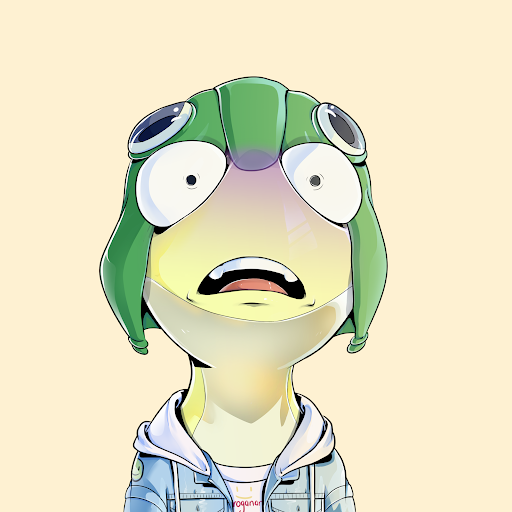
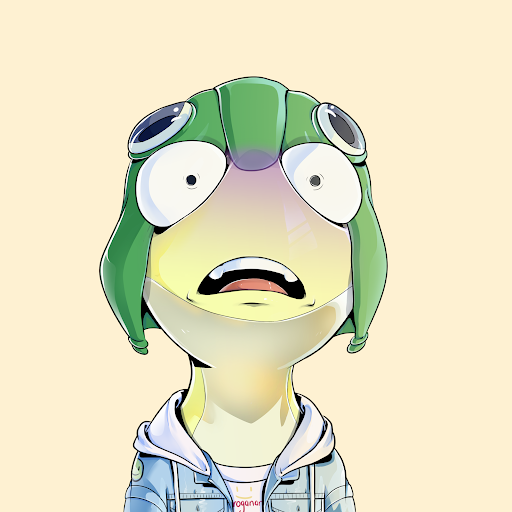
Table of Contents
1. Introduction
2. Problems in the Centralized AI Market
2-1. Excessive market monopoly and data bias
2-2. Data misuse and lack of incentives
3. Bittensor: A Decentralized AI Infrastructure Network Enabling Everyone to Contribute to AI Model Training and Earn Incentives
4. The Introduction of dTAO: A Paradigm Shift in Bittensor’s Tokenomics
4-1. dTAO: Driven by the demand for fair and scalable tokenomics
4-2. dTAO empowers each subnet to possess its own token
4-3. dTAO is expected to drive ecosystem expansion
4-4. dTAO deepens user engagement in the AI ecosystem
4-5. Early-stage challenges of dTAO: Liquidity constraints and potential issues
5. Final Thoughts - When the AI Momentum Returns, Bittensor Will Be a Must-Watch Project
1. Introduction
The emergence of DeepSeek has sent shockwaves through the AI industry. Until now, it was an accepted fact that building a large language model (LLM) required capital expenditures in the billions of dollor. however, DeepSeek claims to have built an LLM comparable to GPT‑o1 for a mere US $6 million. This not only accelerated the AI hegemony war between China and the United States, but also spurred companies and startups—previously deterred by the inability to invest trillions in CAPEX—to venture into building their own models. Paradoxically, this has become the catalyst for greatly expanding the AI model market.
The reduction in costs for building AI models, thereby lowering the entry barrier, presents a significant opportunity for Bittensor. Except for cases where one aims to build the very latest training/inference model to compete with Big Tech, billion of dollor in capital are not necessary. Competitive models can be built with relatively modest funding. By leveraging its subnets, Bittensor can explore a wide range of innovative experiments. Moreover, as various parties develop their own models, we can expect a shift away from a one-size-fits-all GPT to customized AI models tailored to individual purposes. Bittensor’s tokenomics—designed to establish a framework in which diverse participants contribute to AI training, computing power, and data provision while earning incentives—can truly shine in this environment.
The expansion of the AI market is a trend that no one doubts; the only debate is over its speed. The recent DeepSeek incident has served as a catalyst to accelerate that pace. In the burgeoning AI model market, Bittensor—which advocates for decentralized AI models—stands a good chance of capturing a significant market share. Some may even favor utilizing AI models for the public good rather than commercializing them exclusively, in contrast to figures like Sam Altman.
This report examines the potential of Bittensor as an infrastructure that supports decentralized AI model building.
2. Problems in the Centralized AI Market
2-1. Excessive market monopoly and data bias
Concerns about the rapid advancement of AI have been raised for a long time. A telling example is the well-known anecdote involving Elon Musk and OpenAI. Elon Musk repeatedly warned that “if AI develops too quickly, it could pose a threat to humanity,” and, driven by these concerns, he co-founded the non-profit research institute OpenAI with Sam Altman to benefit humanity rather than generate commercial profits. However, as Sam Altman and the management team gradually steered OpenAI toward commercialization, Musk eventually left the company over these differences.
Musk’s concerns are not to be dismissed lightly. Today’s AI market is dominated by a handful of large companies—such as OpenAI, Google DeepMind, and Anthropic—with generative AI technology developed and operated in a highly closed manner. OpenAI and Google now command roughly 75% of the generative AI market share, and most users can access AI functionalities only through the APIs provided by these companies. In such a restricted structure, the benefits of technological advancement become concentrated in a few hands, leaving the overall market under the control of select entities. Can we be confident that if a handful of fintech companies and specific AI models dominate the world, Elon Musk’s fears about AI posing an existential threat will not come true?
Source: Terminator (scene depicting AI deeming humans as threats and engaging in mass slaughter)
Another structural issue is the “long-tail” problem arising from data bias. Generative AI models are trained on vast amounts of data. If this data is skewed toward a particular region, language, or cultural sphere, then the resulting AI judgments will also be biased. In fact, research has shown that while GPT‑4 achieves an accuracy of 86.4% on English-based documents, performance in non-English languages can drop by over 30%. This implies that even though AI services are global, they may operate in ways that favor the interests of specific groups. If a few companies monopolize AI model development and restrict data access, the perspectives of diverse users and minority groups could be excluded from the technology.
2-2. Data misuse and lack of incentives
Currently, in the AI ecosystem, vast amounts of data are being indiscriminately exploited by large AI companies, while the actual contributors to that data receive little to no incentives. AI models are built using data gathered through massive web crawling efforts; yet, in the process, original content creators and data providers often receive no compensation. Whether it’s news articles, blog posts, social media content, academic papers, code, or images, these various forms of content are used for AI model training, but many of the original creators remain unaware that their work is being used.
This issue deepens as AI technologies advance. As models become more sophisticated, the demand for data increases, and more creative works are used like “orphaned assets.” Some artists have even filed lawsuits claiming that AI has used their works without permission to generate outputs, and media outlets have criticized the fact that, despite their content being used for AI training, the original creators receive no incentives. Researchers have similarly noted that although academic papers and technical documents are used for training AI models, the creators of this knowledge do not see any economic benefits.
3. Bittensor: A Decentralized AI Infrastructure Network Enabling Everyone to Contribute to AI Model Training and Earn Incentives
As discussed earlier, the current AI ecosystem is dominated by a handful of large companies that exclusively develop models while exploiting vast amounts of data without providing proper incentives to the data contributors. In such a structure, technological advancements in AI tend to concentrate in the hands of a few, leaving individuals or groups from diverse backgrounds excluded from the ecosystem. In response to these structural limitations, Bittensor emerges as a project aimed at integrating blockchain technology into the AI space—allowing anyone to participate in AI development and earn incentives based on their contributions.
Bittensor is built on a Proof-of-Stake (PoS) blockchain network called Subtensor, which was created using Substrate, the RUST-based open-source blockchain framework developed by Polkadot. Complementing Subtensor are dozens of off-chain networks called subnets. Subtensor evaluates each subnet and records the results on-chain, while each subnet functions as an independent network unit dedicated to specific AI tasks (such as AI training, providing computing power, or supplying data). Anyone can launch a new subnet and operate their own AI model, and anyone can join an existing subnet to perform AI tasks and receive incentives. In practice, the roles of these subnets are categorized into areas such as LLM model development, inference, computing power, AI agent development, and data layers. As of March 22, there are a total of 79 subnets in existence.
Source: backprop.finance
Bittensor’s network architecture centers on a miner-driven subnet structure, with Subtensor functioning as the validator. To illustrate Bittensor’s structure, consider its flagship subnet, Chutes.
Chutes enables anyone to contribute GPU computing power to create and run various AI models. In this context, GPU providers who support Chutes become the subnet’s miners. The validators within Subtensor assess the provided GPU computing power, and the resulting weight—calculated via the YC (Yuma Consensus) algorithm—is used to determine reward distribution fairly. Rewards are paid in TAO, Bittensor’s native token (note that the reward structure underwent some changes following the introduction of dTAO, which will be discussed in Section 4).
The key point in this process is that participation in AI model creation occurs in a decentralized manner—unlike the traditional, big-tech dominated approach—and that incentives are allocated transparently through the YC algorithm. Specifically, 41% of rewards are allocated to miners (the subnet contributors), another 41% to validators on Subtensor, and the remaining 18% to subnet operators.
To delve a bit deeper into representative subnets, let’s look at Chutes and Targon.
Currently, several models—including DeepSeek-R1—are running on Chutes, and through fine-tuning, a range of specialized AI models for applications such as trading and image generation are being offered. For instance, when using DeepSeek-R1 on Chutes, the cost is approximately $2.19 per 1 million tokens processed (roughly 750,000 words). While DeepSeek-R1 is open-source and can be run by anyone, the costs vary depending on the execution environment (local GPU, Chutes, or cloud infrastructure like AWS), generally ranging from $1 to $15 per 1 million tokens. In this regard, Chutes presents a highly cost-effective solution.
Moreover, DeepSeek-R1 demonstrates performance close to that of GPT-4, and has proven competitive across various benchmarks. In contrast, calling GPT-4 on the same basis (per 1 million tokens) costs about $40, making model execution via Chutes a compelling choice in terms of both cost and performance. This clearly illustrates the efficiency and future potential of building AI models on the Bittensor subnets.
Source: Chutes (various AI models running on Chutes)
Targon, on the other hand, is a decentralized AI inference subnet that supports a variety of high-performance open-source LLMs, making them available via APIs for anyone to use. Targon’s miners employ high-performance GPUs to execute LLM inference and are evaluated by validators based on performance and consistency. Users can access models deployed on Targon via API calls, or even lease a specific model on a weekly basis to power their own AI services.
Targon’s primary goal is to deliver high-performance LLMs. Currently, it supports various models with proven performance, such as DeepSeek-V3, Meta-Llama 3.1, Qwen2.5, and Hermes-3—most of which operate in a high-performance inference environment capable of processing hundreds to thousands of tokens per second. For example, NVIDIA’s Llama-3.1-Nemotron-70B-Instruct
model has recorded speeds of up to 2,322 TPS (tokens per second), and DeepSeek-V3 similarly processes in a large cluster powered by 16 GPUs.
Because of this architecture, Targon’s costs are somewhat higher compared to other LLM platforms. Models like Hermes-3 and Qwen2.5 can be leased for around $250 per week, while high-performance models such as Llama-3.1-Nemotron command rates of approximately $1,000 per week. Although these costs might seem high on a per-unit basis, the fixed weekly fee combined with high TPS and unlimited API calls makes Targon a more cost-effective solution in environments that require high traffic and continuous service.
Targon’s performance and architecture are made possible by the Bittensor subnet. The validator–miner structure has fostered a competitive ecosystem focused on performance and accuracy, and the incentive distribution system based on the Yuma Consensus drives miners to optimize both speed and precision. Moreover, the decentralized GPU network—which anyone can join—ensures both flexibility and scalability.
Source: Targon
This goes beyond mere differences in incentive mechanisms; it represents a fundamental shift that distributes opportunities and authority for AI development among a wide range of participants. Unlike previous models that relied on unauthorized use of crawled internet data or internal assets of large corporations, Bittensor is designed to ensure that economic value is returned to the actual data providers. As a result, TAO functions not merely as a token, but as a unit of value that fairly quantifies the contributions of diverse participants in the AI ecosystem. This is critical to lowering the barriers to technological participation and balancing the data production–consumption flow.
Despite this robust structure, some issues surfaced. In response, Bittensor revamped its tokenomics and architecture in February by introducing dTAO. Section 4 will explore the problems that emerged from the previous structure and delve into dTAO in greater detail.
4. The Introduction of dTAO: A Paradigm Shift in Bittensor’s Tokenomics
4-1. dTAO: Driven by the demand for fair and scalable tokenomics
The TAO-based tokenomics model and Subtensor architecture described earlier were effective in the early stages. When there were only a few subnets, validators could evaluate them relatively fairly, and a trusted group of validators maintained network balance through equitable incentive distribution. However, as the number of subnets grew and the ecosystem expanded, the limitations of the validator-based incentive system began to emerge.
The first issue was that it became practically impossible for validators to assess all subnets fairly, which hindered scalability. As the number of subnets increased, validators tended to assign more weight to those they were already familiar with, making it difficult for new subnets to gain traction. This resulted in higher entry barriers between subnets and a decline in network diversity.
The second issue was the potential for collusion between validators and subnet operators. Although the YC (Yuma Consensus) algorithm filtered out some discrepancies, validators still had the ability to favor certain subnets. Because incentives were determined by validators’ subjective assessments, the performance of the subnets that actually conducted AI computations was not necessarily aligned with the incentives awarded. In such a structure, relationships and weight distribution within the network could outweigh actual performance, leading to inefficient resource allocation across the system.
The third issue was that miners and subnet operators lacked sufficient incentives to hold TAO. In order to cover operating expenses, these participants sold off TAO, continuously exerting downward pressure on its price.
To address these challenges and establish a more efficient and equitable tokenomics structure, Bittensor introduced Dynamic TAO (dTAO). dTAO shifts the TAO incentive mechanism from a validator-vote-based system to a market-driven, auto-adjusting model that promotes healthy competition between subnets, allowing the network to operate more autonomously and transparently.
4-2. dTAO empowers each subnet to possess its own token
The most significant change brought by dTAO is the transition from a validator-vote-based TAO incentive mechanism to one based on market pricing. Previously, validators would assess subnets and allocate TAO according to assigned weights, but now each subnet’s economic value is directly determined by market pricing, which then forms the basis for incentive distribution.
To achieve this, dTAO abandons the single-token TAO model in favor of a “one subnet, one token” approach. Under the previous system, all subnets used the same TAO and incentives were determined solely by validator assessments. With dTAO, each subnet issues its own subnet token (called Alpha), and the price of this token reflects the subnet’s performance. Incentives are distributed differentially based on the market price of the subnet token.
Source: Taostats (Token list of subnets)
Users who believe a particular subnet has strong growth potential can stake TAO to acquire that subnet’s Alpha token, ensuring that the subnet’s economic value is more accurately represented within the network. To facilitate automated price adjustments, dTAO incorporates an Automated Market Maker (AMM)-based liquidity pool. The price of each subnet’s Alpha token is determined by the scale of TAO staked in that subnet, with higher TAO stakes driving up the Alpha token price.
Source: Bittensor (Price of Alpha tokens)
The TAO incentive mechanism has also been completely transformed. The higher the Alpha token price for a given subnet, the greater the incentives(Alpha) it receives. Like TAO, Alpha tokens have a fixed total supply of 21 million and experience periodic halvings to reduce emission rates. The incentives distributed from newly minted Alpha are allocated as follows: 50% to the liquidity pool, 20.5% to miners, 20.5% to validators, and 9% to subnet operators.
However, this structure also carries the risk that a small number of participants (whales) with substantial capital might artificially inflate Alpha prices and monopolize incentives. To counteract this, the dTAO model includes a mechanism that reduces token issuance when Alpha prices spike, thereby preventing any single subnet’s incentives from becoming disproportionately unbalanced in the market.
4-3. dTAO is expected to drive ecosystem expansion
dTAO does more than simply alter the incentive mechanism—it is poised to serve as a catalyst for ecosystem expansion within the Bittensor network. With dTAO, the economic value of each subnet is evaluated directly by the market, creating an environment where competition between subnets occurs more autonomously.
This change provides stronger incentives for subnet operators. To earn higher incentives, subnet operators now need to boost their Alpha token price rather than rely solely on subjective validator assessments. In other words, subnet operators must continually improve the performance of their AI models and attract more users. As competition among subnets becomes more balanced under dTAO, overall innovation and development across the network are expected to accelerate.
Moreover, the network’s scalability is likely to improve. Under the previous model, validator assessments could serve as a barrier for new subnets entering the network. With dTAO, market participants directly evaluate subnet value and allocate resources accordingly, allowing new subnets to quickly gain traction and stimulating more vigorous competition among subnets. This environment is expected to foster increased diversity in AI research and development, enabling new technologies and models to be tested and implemented freely.
4-4. dTAO deepens user engagement in the AI ecosystem
The introduction of dTAO marks a shift in the operational model of the AI network toward a more community-centric approach. Previously, users primarily played a passive role in consuming AI models, but dTAO transforms them into active participants in the economic decision-making process of the AI network.
Under the old validator-based incentive model, decision-making power was concentrated among validators. dTAO, by contrast, evaluates the value of AI models based on market prices rather than subjective judgments, enabling users to have a direct impact on the network’s economy. Users can buy and stake the subnet tokens they deem valuable, thereby influencing the growth direction and incentive emissions of that subnet.
This community-driven structure tightens the relationship between AI model developers and users, reinforcing the autonomy and sustainability of the AI ecosystem. Through direct contribution and economic incentives, users can help build a more efficient, user-centric AI ecosystem. This represents a fundamental shift beyond mere token mechanics—creating an environment where AI technology is driven by community participation.
4-5. Early-stage challenges of dTAO: Liquidity constraints and potential issues
In the initial stages of dTAO implementation, several structural challenges have emerged. The new model, in which the price of the subnet token (Alpha) determines incentives, is more autonomous and fair—but it is not without its imperfections. A disconnect between the objective performance or value of an AI model and its token price may occur. In practice, even if an AI model demonstrates high performance or sees active usage, projects with low initial liquidity or weak marketing may see their token prices underperform. Conversely, if a small group of whales or early community influencers artificially pumps the token price, a subnet could receive excessive incentives without having sufficient technical capability or genuine user engagement. This could distort the incentive structure through speculative market flows.
Furthermore, in the dTAO framework, prices are determined by the liquidity available in TAO and Alpha tokens. During the early launch phase, when liquidity is relatively low, price volatility can be high. As a result, it is possible to artificially pump a subnet’s token price through TAO staking in the initial phase. In fact, there have been cases where anonymous users exploited Bittensor’s subnets as a platform for launching meme coins. Although Bittensor is transitioning from the old model to dTAO gradually to mitigate such abuses, vigilance remains necessary.
On the positive side, linking the Alpha token price directly to incentives provides a natural incentive for subnet stakeholders—miners and operators alike—to refrain from excessive selling. This encourages long-term price maintenance and helps curb unnecessary token dumping, contributing to overall liquidity stability across the network.
In one case, a subnet was treated like a meme coin, leading to an approximate 98% price drop after intervention by the Bittensor Foundation. (Source: taostats)
5. Final Thoughts - When the AI Momentum Returns, Bittensor Will Be a Must-Watch Project
As mentioned in the introduction, the AI momentum is no longer a matter of debate—it is a clear direction. In today’s crypto market, various initiatives such as AI infrastructure, AI agents, and DeFAI are emerging, rapidly evolving on both technological and tokenomics fronts. In this dynamic landscape, I believe that if AI momentum re-emerges, the first area to capture attention will be infrastructure projects. History from the IT industry shows that explosive application adoption can only occur after the underlying infrastructure has matured. Without a stable and scalable infrastructure, no killer AI application can be sustainable. Therefore, at a time when the integration of AI and blockchain is being actively explored, it is essential to focus on projects that are making significant strides in AI infrastructure.
In this context, Bittensor occupies a unique position in designing and realizing decentralized AI infrastructure. Through its subnet structure, Bittensor provides an environment where anyone can create, evaluate, and earn incentives for their AI models. Its adoption of dTAO strives to achieve both fairness and scalability. Notably, the model in which each subnet has its own unique token goes beyond mere decentralization—it enables token-based community participation and grants the network true economic autonomy. Ultimately, what we must focus on is not merely the growth of AI networks, but the underlying infrastructure that will support that growth—and at the heart of that infrastructure are projects like Bittensor. I conclude with the expectation that Bittensor will expand its subnet ecosystem through the dTAO structure, paving the way for a robust and sustainable future.